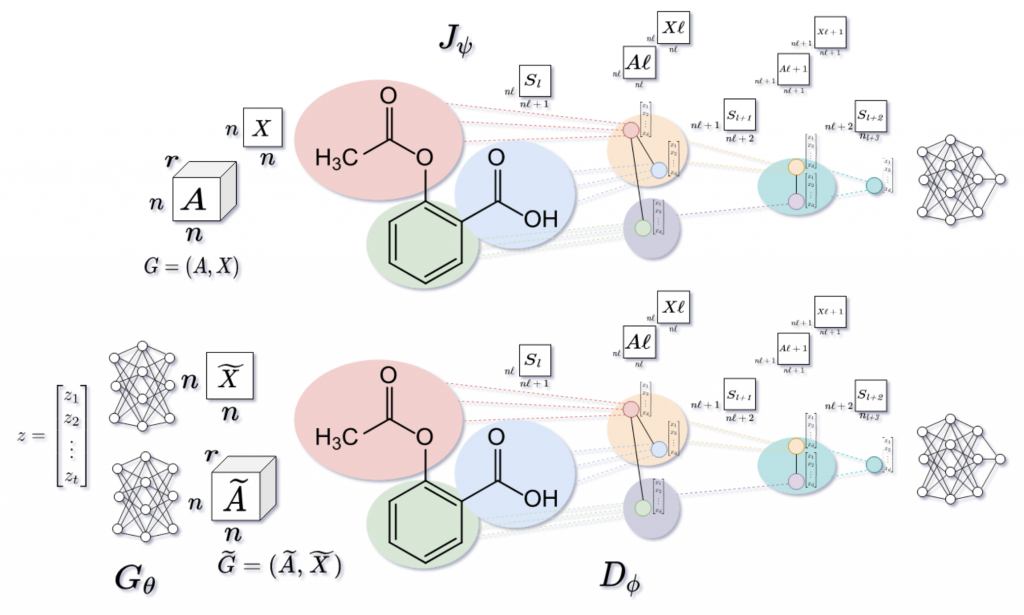
Five years ago, a groundbreaking paper introduced DiPol-GAN, a generative adversarial network (GAN) designed to create molecular graphs with specific chemical properties. Authored by Michael Guarino, et al. in 2019, this work remains a testament to the ingenuity at the intersection of machine learning and computational chemistry. Let’s dive into its contributions and why it continues to be influential.
From Molecules to Meaning: DiPol-GAN’s Key Innovations
DiPol-GAN broke new ground by addressing the complexities of molecular graph generation—a task requiring precision and adherence to chemical constraints. Here are the standout elements of this innovative approach:
- Direct Graph Representations
While many models relied on SMILES strings to describe molecular structures, DiPol-GAN embraced the inherent richness of graph representations. Nodes represented atoms, edges captured bonds, and the graph structure preserved the relational nature of molecules—key for meaningful chemical property optimization. - Hierarchical Reasoning with DIFFPOOL
The paper introduced the use of Differentiable Pooling (DIFFPOOL) in the GAN discriminator. DIFFPOOL hierarchically aggregated graph nodes, allowing the model to extract high-level features and improve classification performance. Moreover, the authors adapted DIFFPOOL to handle multi-relational graphs, capturing the nuances of bond types—an essential feature for molecular modeling. - Reinforcement Learning for Targeted Molecules
DiPol-GAN incorporated a policy network to nudge the generative process toward molecules with desired properties, like solubility (logP) or drug-likeness. This clever integration of reinforcement learning allowed the model to focus on chemically relevant outcomes, setting a precedent for property-driven molecular design.
Five Years Later: Why DiPol-GAN Still Resonates
Even as research in graph neural networks and molecular AI progresses, DiPol-GAN’s contributions remain strikingly relevant:
- Raising the Bar for Molecular GANs: By addressing the dual challenges of graph isomorphism and multi-relational edges, this model set a high standard for graph-based GANs.
- Chemical Property Alignment: The integration of reinforcement learning into graph generation directly inspired modern approaches to property-targeted molecule design.
- Benchmark Metrics: The study’s rigorous evaluation on validity, uniqueness, and novelty using the QM9 dataset provided benchmarks that still guide research today.
Continuing the Journey
DiPol-GAN’s legacy reminds us of the powerful synergy between machine learning and chemistry. Whether you’re exploring novel graph neural network architectures or advancing property-driven molecule generation, this paper offers invaluable insights.
For those working in related domains, revisiting this milestone study could spark new ideas and breakthroughs. Be sure to include it in your references to honor its influence and acknowledge the innovation it brought to the field:
Guarino, M., & Shah, A. Rivas, P., (2019). DiPol-GAN: Generating Molecular Graphs Adversarially with Relational Differentiable Pooling. Presented at the LXAI Workshop @ Neural Information Processing Society Conference (NeurIPS), pp. 9. [download]
Let’s keep building on this foundation, advancing science one molecule at a time.
