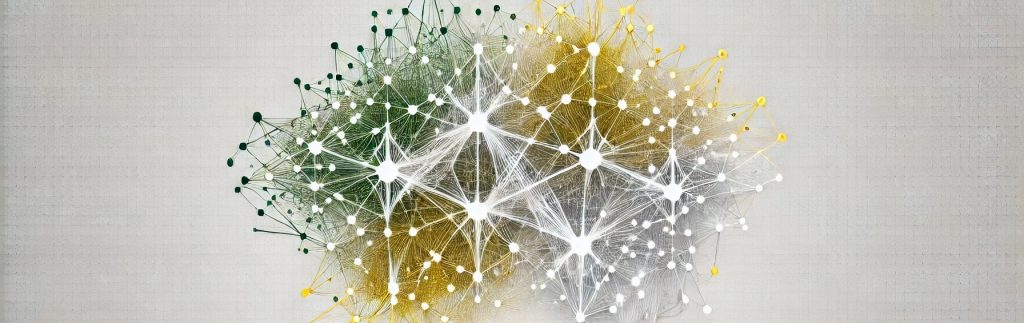
Artificial intelligence (AI) is now embedded in everyday life, from self-driving cars to medical diagnostic tools, enabling tasks to be performed faster and, in some cases, more accurately than humans. However, this rapid advancement comes with significant challenges, particularly in the form of adversarial attacks. These attacks exploit small, often imperceptible changes in input data to deceive AI systems into making incorrect decisions. For example, a strategically placed sticker on a stop sign might cause an AI-powered car to misinterpret it as a speed limit sign, creating potentially dangerous situations; another example can be small perturbations added to your dog’s picture, which can lead to state-of-the-art AI to confuse it with a cat:

The Role of ReLU and Its Limitations
The Rectified Linear Unit (ReLU) activation function is a foundational component of many AI models. Its simplicity and efficiency have made it a go-to choice for training deep learning networks. However, ReLU’s unrestricted output can make models vulnerable to adversarial noise, leading to cascading errors in predictions. Attempts to address this vulnerability, such as Static-Max-Value ReLU (S-ReLU or capped ReLU), have introduced fixed output caps, but these solutions often underperform on more complex datasets and tasks.

Introducing D-ReLU
D-ReLU represents a significant advancement over traditional ReLU. It incorporates a dynamic output cap that adjusts based on the data flowing through the network. This adaptability serves as a robust defense mechanism against adversarial inputs while maintaining computational efficiency. In essence, D-ReLU acts as a self-adjusting safeguard, preserving model integrity even under duress.
Key Features of D-ReLU:
- Adaptive Output Limits: D-ReLU employs learnable caps that evolve during training, enabling models to balance robustness and accuracy effectively.
- Enhanced Resilience: D-ReLU has demonstrated superior performance against adversarial attacks, including FGSM, PGD, and Carlini-Wagner, while maintaining consistent performance on standard datasets.
- Scalability: Tested on large-scale datasets like CIFAR-10, CIFAR-100, and TinyImagenet, D-ReLU has proven its ability to scale effectively without degradation in performance.
- Efficient Training: Unlike adversarial training methods, which require extensive additional computations, D-ReLU achieves robustness naturally, streamlining the training process.
- Real-World Viability: D-ReLU excels in real-world scenarios, including black-box attack settings where attackers lack full knowledge of the model.

The Broader Implications
In applications where reliability and safety are paramount—such as autonomous vehicles, financial systems, and medical imaging—D-ReLU offers a compelling solution to the challenges posed by adversarial inputs. By enhancing a model’s resilience without sacrificing performance, D-ReLU provides a vital upgrade for AI systems operating in high-stakes environments.
Future Directions
The potential of D-ReLU extends beyond current implementations. Areas of exploration include:
- Further optimization for improved performance,
- Applications in natural language processing and audio tasks,
- Integration with complementary robust training methods for enhanced results.
For a detailed analysis and technical insights, download our paper here. If you are working on AI models, we encourage you to experiment with D-ReLU and share your experiences:
Sooksatra, Korn, and Pablo Rivas. 2024. “Dynamic-Max-Value ReLU Functions for Adversarially Robust Machine Learning Models” Mathematics 12, no. 22: 3551. https://doi.org/10.3390/math12223551
About the Author
Korn Sooksatra is a Ph.D. student at Baylor University, specializing in adversarial machine learning and AI robustness.