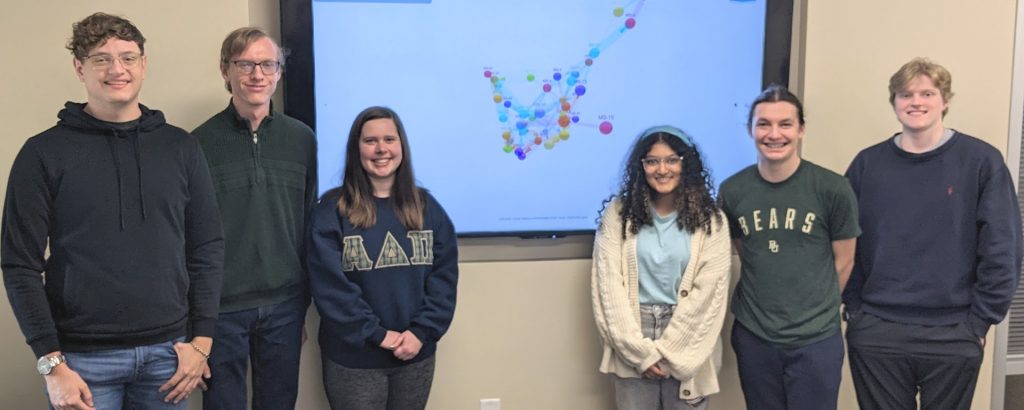
Baylor.AI lab is thrilled to announce the launch of a new research stage in our project that uses NLP to identify suspicious transactions in omnichannel online C2C marketplaces. Under the guidance of Dr. Pablo Rivas and Dr. Tomas Cerny, two groups of undergraduate students participating in NSF’s REU program will contribute to this exciting project.
Misty and Andrew, under the direction of Dr. Pablo Rivas, will be working on designing data collection strategies for the project. Their goal is to gather relevant information to support the research and ensure the accuracy of the findings.
Meanwhile, under Dr. Tomas Cerny’s direction, Patrick, Mia, Austin, and Garrett will focus on data visualization and large graph understanding. Their role is crucial in helping to understand and interpret the data collected so far.
The research project investigates the feasibility of automating the detection of illegal goods or services within online marketplaces. As more people turn to online marketplaces for buying and selling goods and services, it is becoming increasingly important to ensure the safety of these transactions.
The project will first analyze the text of online advertisements and marketplace policies to identify indicators of suspicious activity. The findings will then be adapted to a specific context – locating stolen motor vehicle parts advertised via online marketplaces – to determine general ways to identify signals of illegal online sales.
The project brings together the expertise of computer science, criminology, and information systems to analyze online marketplace technology platform policies and identify platform features and policies that make platforms more vulnerable to criminal activity. Using this information, the researchers will generate and train deep learning-based language models to detect illicit online commerce. The models will then be applied to markets for motor vehicle parts to assess their effectiveness.
This research project represents a significant step forward in the fight against illegal activities within online marketplaces. The project results will provide law enforcement agencies and online marketplaces with valuable insights and evidence to help them crack down on illicit goods or services sold on their platforms.
We are incredibly excited to see what Misty, Andrew, Patrick, Mia, Austin, and Garret will accomplish through this project. We can’t wait to see their impact on online criminal activity research. Stay tuned for updates on their progress and more information about this cutting-edge project.
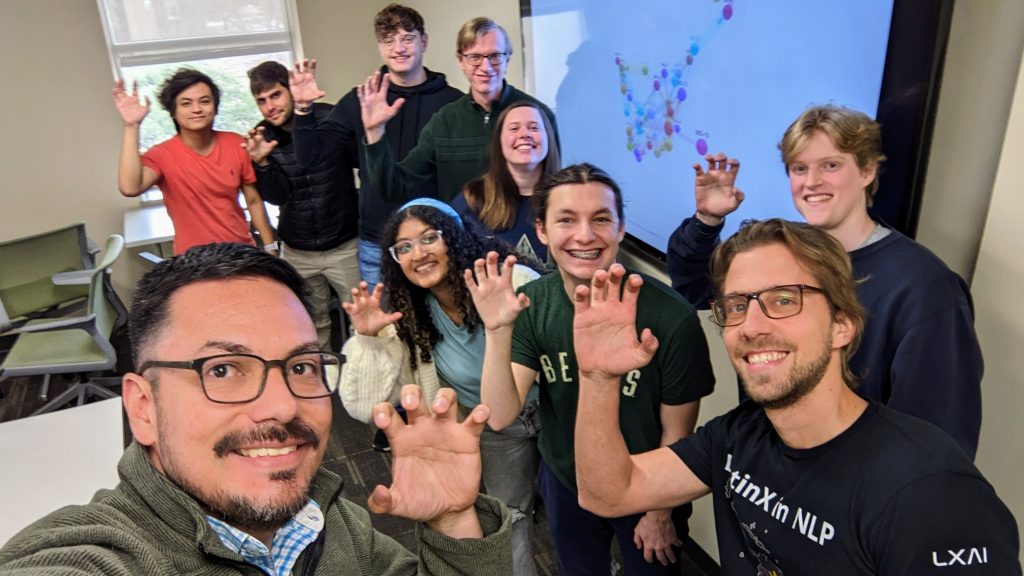