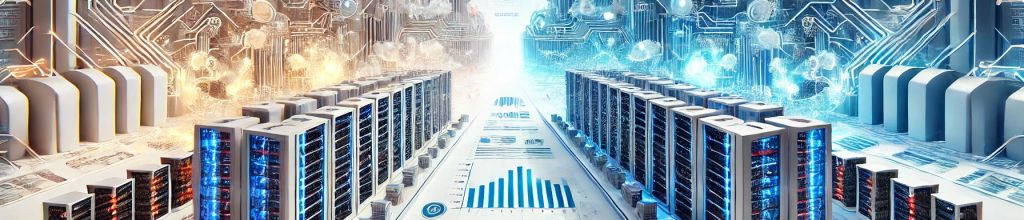
In the world of artificial intelligence, the race for dominance has largely been dictated by computational power. The prevailing logic: the more GPUs, the bigger the dataset, the stronger the model. But what if this assumption is fundamentally flawed?
DeepSeek, a rising AI startup out of China, is challenging this notion, promising cutting-edge models that rival OpenAI, Anthropic, and Meta—all while operating at a fraction of the cost. Their success raises a number of critical questions. Is DeepSeek proving that AI development has been artificially expensive? Or are their cost-saving claims exaggerated? And most importantly, is this kind of efficiency a win for AI progress, or does it introduce risks that we aren’t fully prepared to address?
The DeepSeek Approach: Scaling Smarter, Not Harder
DeepSeek’s key selling point is efficiency. Rather than relying on the brute-force hardware scaling seen in the West, DeepSeek claims to prioritize smarter architectures and leaner training methodologies.
Take their DeepSeek-R1 model, released in January 2025. It reportedly performs at the level of OpenAI’s top-tier reasoning models, yet was trained at just $5.6 million—a staggering 95% cost reduction compared to OpenAI’s estimated $100+ million in training costs. (VentureBeat)
However, AI experts like Dario Amodei (CEO of Anthropic) have raised doubts about these figures. While DeepSeek may have only spent $5.6 million on the final training run, the total cost—including research, failed experiments, and data collection—likely mirrors what U.S. companies spend. The question remains: is DeepSeek truly disrupting AI economics, or are they merely presenting a selective version of their costs? (Skepticism on the cost figures)
Even if DeepSeek’s claims are valid, what are the trade-offs of this efficiency? If AI models can be developed at dramatically lower costs, we must consider what happens when bad actors gain access to cutting-edge AI capabilities with minimal financial barriers.
DeepSeek’s Market Disruption: A Warning Shot to Western AI Labs?
DeepSeek’s rapid success is already rattling the AI industry.
- Their DeepSeek-V3 model, a 671-billion-parameter behemoth, is now being compared to OpenAI’s GPT-4o and Meta’s LLaMA 3.1.
- Their app has overtaken ChatGPT as the top-ranked AI app in multiple countries. (TechCrunch)
- NVIDIA, long assumed to be the main benefactor of the AI boom, saw a 17% stock drop as investors questioned whether raw GPU power is still the main driver of AI progress. (New York Times)
If DeepSeek can achieve competitive AI models at lower costs, what does this mean for companies like OpenAI, Google DeepMind, and Anthropic, which rely on massive cloud computing investments?
The Geopolitical Angle: Is the West’s AI Strategy Backfiring?
DeepSeek’s success also exposes the limitations of U.S. export controls on AI technology.
- The U.S. government has imposed strict restrictions on high-end chips being sold to China, attempting to slow down AI progress.
- However, instead of stalling Chinese AI development, these restrictions may have forced China to innovate more efficiently.
Recent reports suggest that Washington may introduce new curbs on NVIDIA chip sales, but is this just an arms race that China has already learned to bypass? (Brookings Institution on export controls)
This raises a difficult question for policymakers: Can AI progress actually be contained? Or will attempts to suppress it simply accelerate the development of alternative methods?
The “Open Source” Illusion: How Open Is DeepSeek?
DeepSeek markets itself as an open-source AI company—but does it truly adhere to open-source principles?
- They have released model weights and architectures, but have not disclosed their training data.
- Researchers like Timnit Gebru argue that “open source” should require full transparency about what data was used to train these models. Otherwise, we have no way of knowing whether they contain harmful biases, stolen intellectual property, or state-approved censorship. (Timnit Gebru’s critique)
Beyond data transparency, there are concerns that DeepSeek’s models are not truly free from government oversight.
- Topics like Tiananmen Square and Uyghur human rights are systematically censored.
- Some users are already finding workarounds to bypass these filters using modified text prompts. (Matt Konwiser’s LinkedIn discussion)
- The open-source AI community is actively reverse-engineering DeepSeek’s models to assess their capabilities and limitations. (Hugging Face’s DeepSeek R1 analysis)
This presents an ethical dilemma: Is an AI model truly open-source if it comes with built-in censorship? If DeepSeek models are widely adopted, will this lead to a fragmented internet where AI tools reinforce state-controlled narratives?
The Regulatory Debate: Can We Trust AI Without Oversight?
DeepSeek’s rise brings us back to a fundamental question: Should AI development be strictly regulated, or should innovation be allowed to run unchecked?
- A recent poll showed that 52% of respondents favor mandatory registration of AI agents to improve transparency.
- There is also strong support for third-party audits and developer accountability.
However, even as AI companies push for more regulation in principle, they often resist the specifics.
- OpenAI recently launched ChatGPT Gov, a version tailored for government use with additional safeguards. (OpenAI’s announcement)
- Meanwhile, Meta is scrambling to justify its costly AI development after DeepSeek’s open-source approach exposed how overpriced corporate AI may be. (Meta’s reaction)
As AI capabilities continue to expand, governments must decide how much control is necessary to prevent misuse while still encouraging innovation.
Final Thoughts: A Paradigm Shift in AI, or Just Hype?
DeepSeek represents a turning point in AI development, but whether this shift is sustainable—or even desirable—remains an open question.
- If DeepSeek’s efficiency claims hold up, Western AI companies may need to rethink their business models.
- If U.S. export controls are failing, new policy approaches will be needed.
- If DeepSeek is truly open-source, AI censorship and data transparency must be scrutinized.
Perhaps the most pressing question is: If AI can now be developed at a fraction of the cost, does this mean an explosion of beneficial AI—or an influx of cheap, powerful AI in the hands of bad actors?
One thing is certain: the AI landscape is shifting, and it’s time to rethink the assumptions that have guided it so far.
– Dr. Pablo Rivas
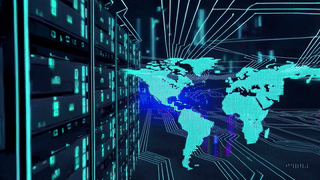